CourtVision: Highlight Your
Key Moments with Machine Learning
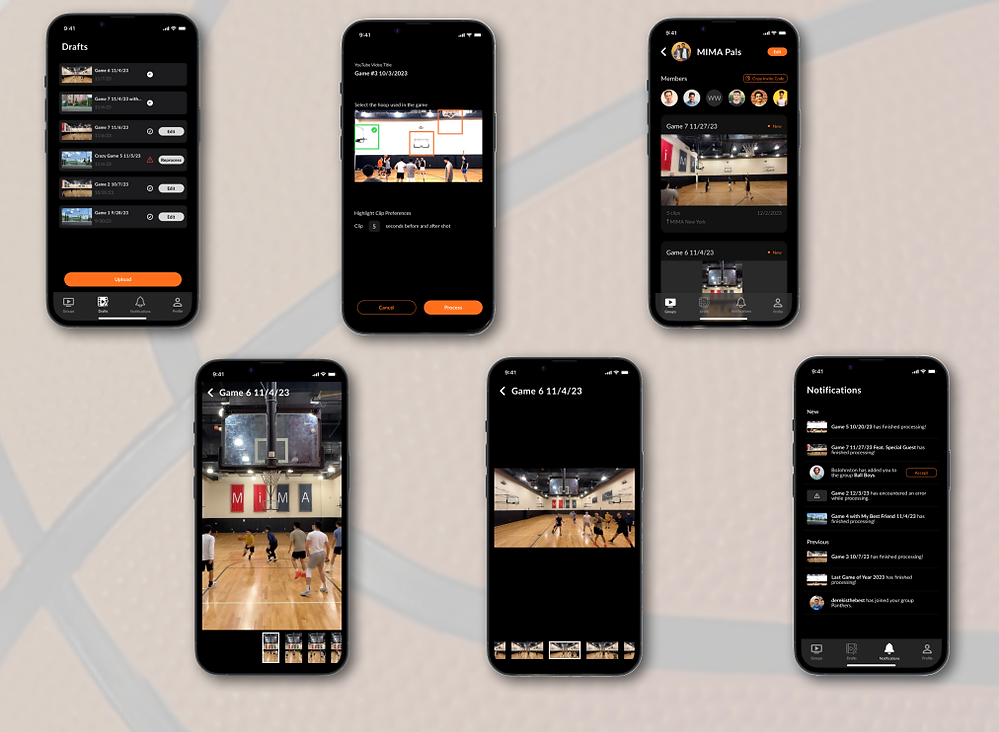
CourtVision leverages ML to distill often long and difficult to share pickup basketball footage to key clips, and provides a platform on which to easily edit and share clips with friends. Started by a group of pals who love to play pickup together, CourtVision seeks to help players improve their basketball on-court skills, as well as deepen friendships between players by sharing key on-court memories.
Client: CourtVision
My Role: Product Designer
Team: 2 Designers, 3 Engineers,
1 Business Lead
Duration: Ongoing
Mission: Research and End-to-End Design
Tools Used: Figma, FigJam, Jira, Photoshop, Google Workspace
OUR CHALLENGE
Confirm problem space and create a tool for amateur pickup basketball players to:
-
Distill their pickup footage into key moments using machine learning
-
Share said clips with their community to deepen on and off-court relationships
SOLUTION
​An intuitive tool that streamlines the upload, analyze and share processes.
-
Guides users through the process of analyzing their often-long pickup footage with machine learning
-
Shares clips via private Groups so that users can easily look back on memorable moments with friends
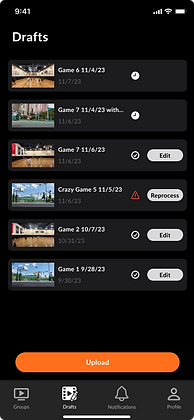

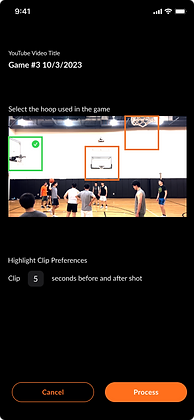

UNIQUE VALUE PROPOSITION

Slash editing and sharing time by hours leveraging machine learning

One of the first (if any) basketballl upskilling tools for amateur basketball players

Create and deepen IRL relationships between players through pickup
How It Started
OUR STORY
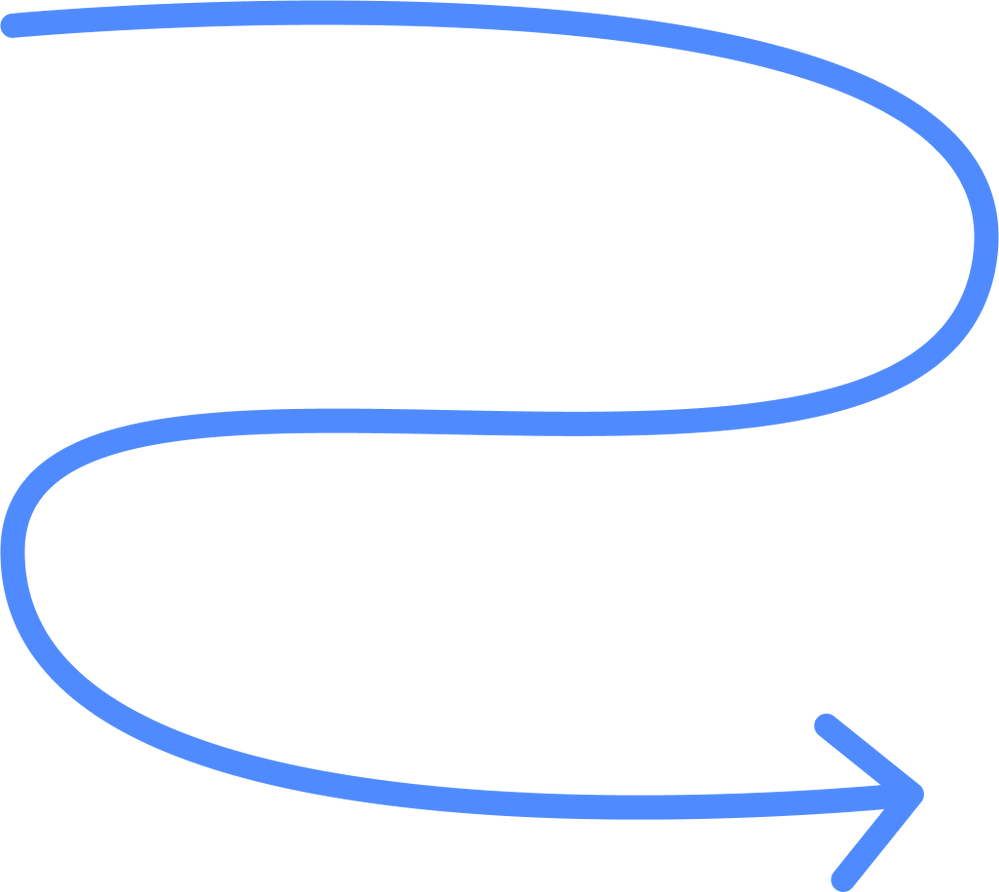
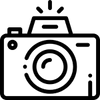
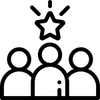
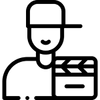



It all started with a group of pals who love to play pickup basketball together.
Out of a want to improve his form and skills, one of the pals, Jon, began filming his pickup games.
Eventually, Jon became the designated “filmer” of his group, and began sending his friends the footage he took of their games.
However, Jon soon began to run into some problems:
-
Each game was 10-15 minutes long, and Jon and his pals played anywhere from 2-5 games per meet up. Trying to send this many large video files could take hours.
-
Finding and trimming each video to key moments (baskets made or almost made, trick shots, funny moments, etc) was time consuming and tedious.
Jon and his friends, who all happened to work in tech, found machine learning codes that allowed them to extract the time stamps of every time a basket was made. Jon and his friends decided to make a tool that incorproates this code as well as make sharing the videos easier.
My co-designer and I were invited on to the project to conduct research and design the UI of the product.
My co-designer and I were invited on to the project to conduct research and design the UI of the product.
CHALLENGE
Our MVP Mission
How to design an easy-to-use interface that is relatively easy to code for part-time engineers that leaves room for growth
​CONSTRAINTS
The Lines to Color Within
How to design an easy-to-use interface that is relatively easy to code for part-time engineers that leaves room for growth

​Team was working on a part-time basis and Business Team's priority was for a swift timeline, so designs had to be simple and efficient to code in a timely manner

Our Engineering team is relatively small and have less than 5 years experience, so communication on what features were feasible with the above timeline was critical

​Because we were already anticipating additional features beyond the MVP, our MVP had to have room for growth but also meet user needs in its initial state
RESEARCH
Research Goals
1: Confirm that the problem space found by Jon and his pals was also experienced among a more general audience.
2: Find any new needs to incorporate into our tool
RESEARCH
Methods
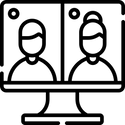.png)
Interviewed amateur players to learn more about what makes a “good game” and what basketball means to them
Interviews
Went to basketball courts around NYC to observe and speak with players in the wild
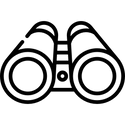
Contextual Inquiry
Analyzed competitor performance-tracking apps and sports-oriented community tools
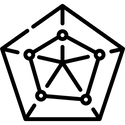
Competitor Analysis
Our Findings
INSIGHTS
​Confirmed Problem Space:






Amateur basketball players who play pickup basketball do take steps to better their skills
The player’s perception of how well they themselves played affected their opinion of how “good” the game was-- if they think they played well, it was a "good" game
Amateur basketball players do film themselves at least occasionally and do share the footage with the other players
If someone sent sent them footage of their game, players would react to/comment on key moments in the footage
New Insights:












Players, often men in their 20s who moved to a new city after college, used basketball as an avenue to meet new friends in addition to get exercise
Basketball was uniquely popular opposed to other sports because of the popularity and infrastructure already present in their cities
After playing a game with someone they didn’t know, asking for their contact to play again was a natural and common occurrence on the court
Teamwork and synergy were equally important in whether a player thought a game was “good” as whether or not they themselves thought they played well
Players tend to film the footage on their phones on landscape mode
Filming can be quite difficult: getting the proper angle to fit everything the player wants in frame is hard, and pressing the record on/off button occasionally moves the phone and therefore shifts what's in frame
There are limited if any performance improvement tools for amateur basketball players
What Made the Cut for MVP?
FEATURE PRIORITIZATION
From the above insights, the design team discovered that in addition to improving skill, building social connection was also a very strong theme among players who routinely played pickup basketball.
After sharing our insights with the Engineers, we mapped out our priorities for our MVP. With our Problem Space confirmed, we were confident in including the necessary steps for video uploading, editing and sharing. However, because of time and technical restraints, the social element of our tool that we wanted to include as a result of our New Insights would be included in the next round of features in our MVP+.

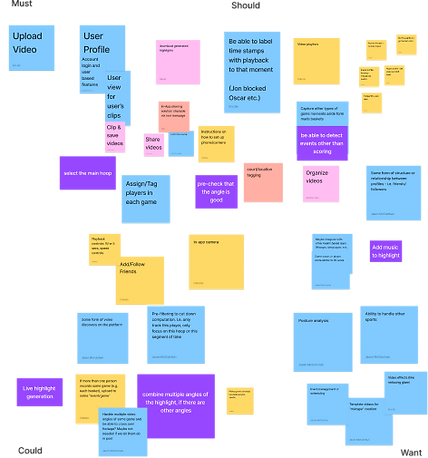

MVP Feature List
FEATURE PRIORITIZATION

Personalize highlight clips by designating how many seconds before and after hoop to keep

Accurate hoop analyzation with hoop and false positive identification and

Easily create and add members to Groups to share game footage

Watch landscape and portrait mode highlight clips
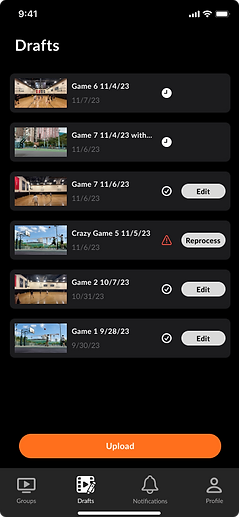

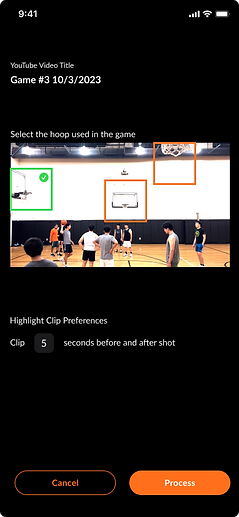

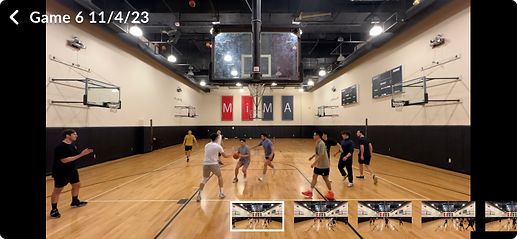
DELIVER
​Technical Documents for Hand-Off
All technical documents were handled in Figma utilizing dev and inspect mode.
​Final file contained Component Library and Screens pages.
​Majority of communication between engineers and designers was done through Slack, Figma comments and annotations on Figma screens.
Annotation Examples

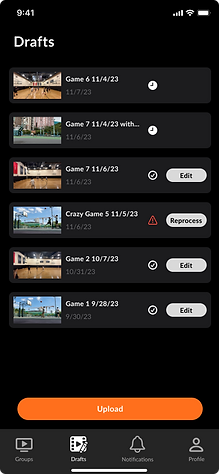
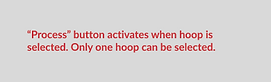
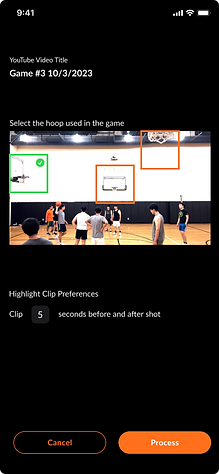
MVP+ Feature List
LOOKING AHEAD
Following the execution of our first version of the MVP, we will be implementing the following features as informed by the design team's research.
Once the MVP is released, CourtVision will expand its capabilities to include connecting amateur pickup basketball players to each other to promote cross-play between users.

Highlight key moments through Comments and reactions

Connect players in local area to build friendships through basketball

​Filming tips on how to film the best footage for analyzation